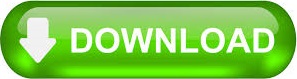
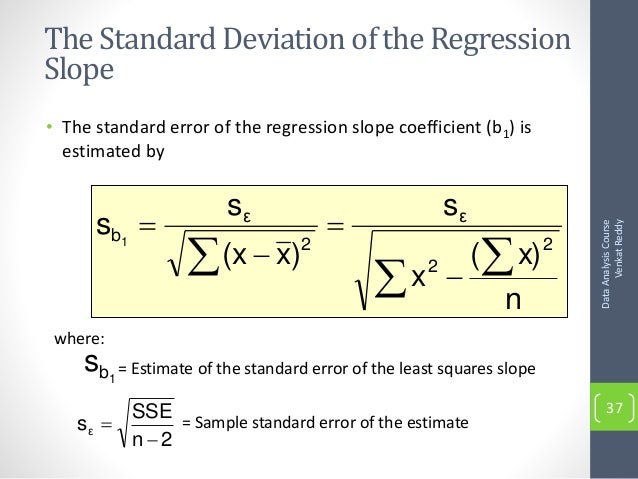
- Standard deviation of linear regression excel serial#
- Standard deviation of linear regression excel manual#
Step 2: Carry Out a Regression Analysis Using the Regression Function Assuming the response is directly proportional to the analyte concentration, you should plot a linear curve. Step 1: Plot a Standard CurveĬonstruct a standard curve by plotting the concentration of analyte on the X-axis and the response on the Y-axis. The SD ( \sigma) can be calculated for the Y-intercept and used to calculate the LOD and LOQ for a standard curve using the regression function in MS Excel (I use version 2013 however, you can use any version and it’ll work just fine) as follows. On the other hand, the calibration curve method using MS Excel is based on the standard deviation (SD) of response ( \sigma) and slope (s) of the calibration curve, thus making it more accurate. However, it may be used to verify the LOD that has been determined via other methods.
Standard deviation of linear regression excel serial#
Thus, in the case of the serial dilution method, there is no exact figure that you can accurately/statistically term as the LOD. Although the dilution subsequent to the assumed LOD (say ‘x’) might not give a response 95% of the time, there is a chance that a concentration between the assumed LOD and ‘x’ might. The serial dilution method defines the LOD as the concentration of analyte that gives you a response 95% of the time across a dilution series. Calculating the LOD and LOQ Using MS ExcelĪmong the methods used to validate analytical methods, such as serial dilutions, computing LOD and LOQ via a calibration curve in MS Excel is more accurate and reliable. Therefore, the standard curve you have freshly plotted requires statistical validation to ensure the responses of your test samples are within the range of detection/quantitation. Furthermore, the sensitivity of every analytical technique differs with the instrument.

For instance, pipetting errors can lead to variations in response when performing serial dilutions to prepare a standard curve.
Standard deviation of linear regression excel manual#
Although you might try your best to avoid incorporating manual errors, there is still a good chance of that happening. It is also wise to compute LOD and LOQ for an already established method. In Validating Established Analytical Methods To ensure that your data are accurate and reliable, it is important to compute the LOD and LOQ for any new analytical technique. If the concentration of the analyte you plan to detect using a certain analytical method that you developed is below the LOD and/or LOQ, then the signal you detect cannot be trusted. For example, HPLTC can be more cost effective and sensitive than the more traditional HPLC. New analytical techniques can have a lot of advantages over tried-and-true methods. The Importance of Computing LOD and LOQ In Validating a New Analytical Method This ensures that the signal is indeed due the analyte, and not due the analytical noise. For this reason, a limit is set for the detection of the analyte (LOD), which is higher than the signals that fall in the analytical noise zone. If the analyte concentration is too low, the analyte signal cannot be distinguished from analytical noise.

When measuring the concentration of an analyte, the analyte must produce a signal greater than the analytical noise, i.e., the signal obtained in the absence of analyte. They are the minimum amount of compound (analyte) that can be reliably detected and quantified, respectively, using an analytical method/instrument. LOD and LOQ mean exactly what these terms suggest. This article will describe what LOD and LOQ are, their importance in method validation, and finally a quick method to calculate these values using Excel. Limit of detection (LOD) and limit of quantitation (LOQ) are the two crucial parameters used to validate analytical methods. Failing to do so, you might end up with erroneous results and waste time and resources. When performing an un-established analytical method, one needs to statistically validate it.
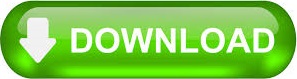